July 2023 - Khan et al. 2023
Streamflow forecasting for the Hunza river basin using ANN, RNN and ANFIS models
Water Practice and Technology. https://doi.org/10.2166/wpt.2023.060
Streamflow forecasting is essential for planning, designing, and managing watershed systems. This research study investigates the use of artificial neural networks (ANN), recurrent neural networks (RNN), and adaptive neuro-fuzzy inference systems (ANFIS) for monthly streamflow forecasting in the Hunza River Basin of Pakistan. Different models were developed using precipitation, temperature, and discharge data. Two statistical performance indicators, i.e., root mean square error (RMSE) and coefficient of determination (R2), were used to assess the performance of machine learning techniques. Based on these performance indicators, the ANN model predicts monthly streamflow more accurately than the RNN and ANFIS models. To assess the performance of the ANN model, three architectures were used, namely 2-1-1, 2-2-1, and 2-3-1. The ANN architecture with a 2-3-1 configuration had higher R2 values of 0.9522 and 0.96998 for the training and testing phases, respectively. For each RNN architecture, three transfer functions were used, namely Tan-sig, Log-sig, and Purelin. The architecture with a 2-1-1 configuration based on tan-sig transfer function performed well in terms of R2 values, which were 0.7838 and 0.8439 for the training and testing phases, respectively. For the ANFIS model, the R2 values were 0.7023 and 0.7538 for both the training and testing phases, respectively.
Tags: ANN, RNN, ANFIS, streamflow, forecasting, precipitation, Hunza river
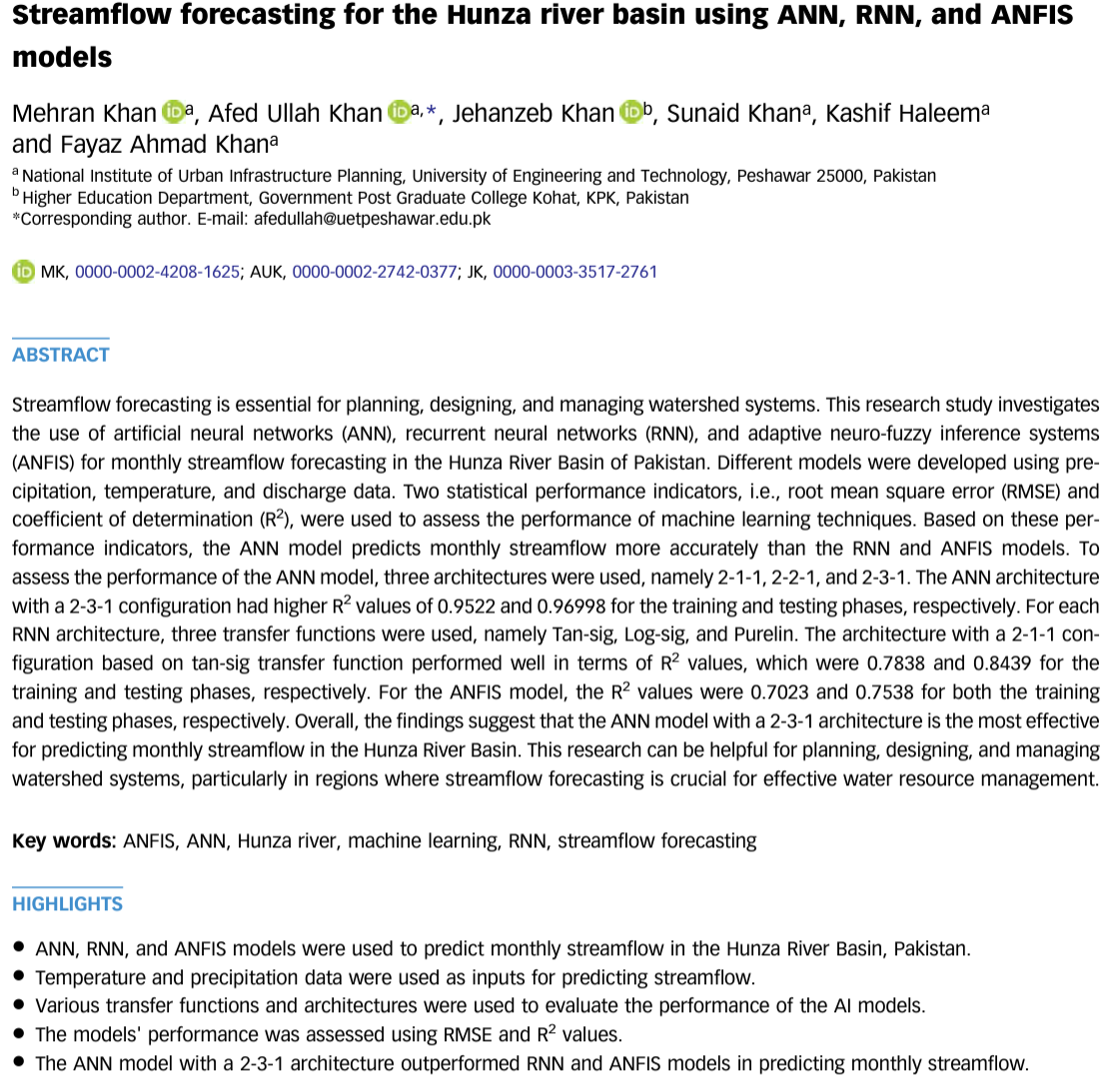